For their paper "Increasing the Performance and Plausibility of Machine Learning via Data Analysis Techniques" Silas Aaron Selzer, Fabian Bauer and Prof. Peter Bretschneider from the Energy Usage Optimization Group have been awarded the Best Paper Award at the 9th International Conference on Time Series and Forecasting (ITISE) 2023 in Meloneras/Gran Canaria. In their article, which will be published in the Springer Verlag textbook "Contributions to Statistics", the scientists use examples from energy technology to show that the use of data analysis techniques for feature selection improves the performance and plausibility of machine learning methods and leads to trustworthy as well as reliable models, which are important for a variety of applications, especially in safety-critical areas.
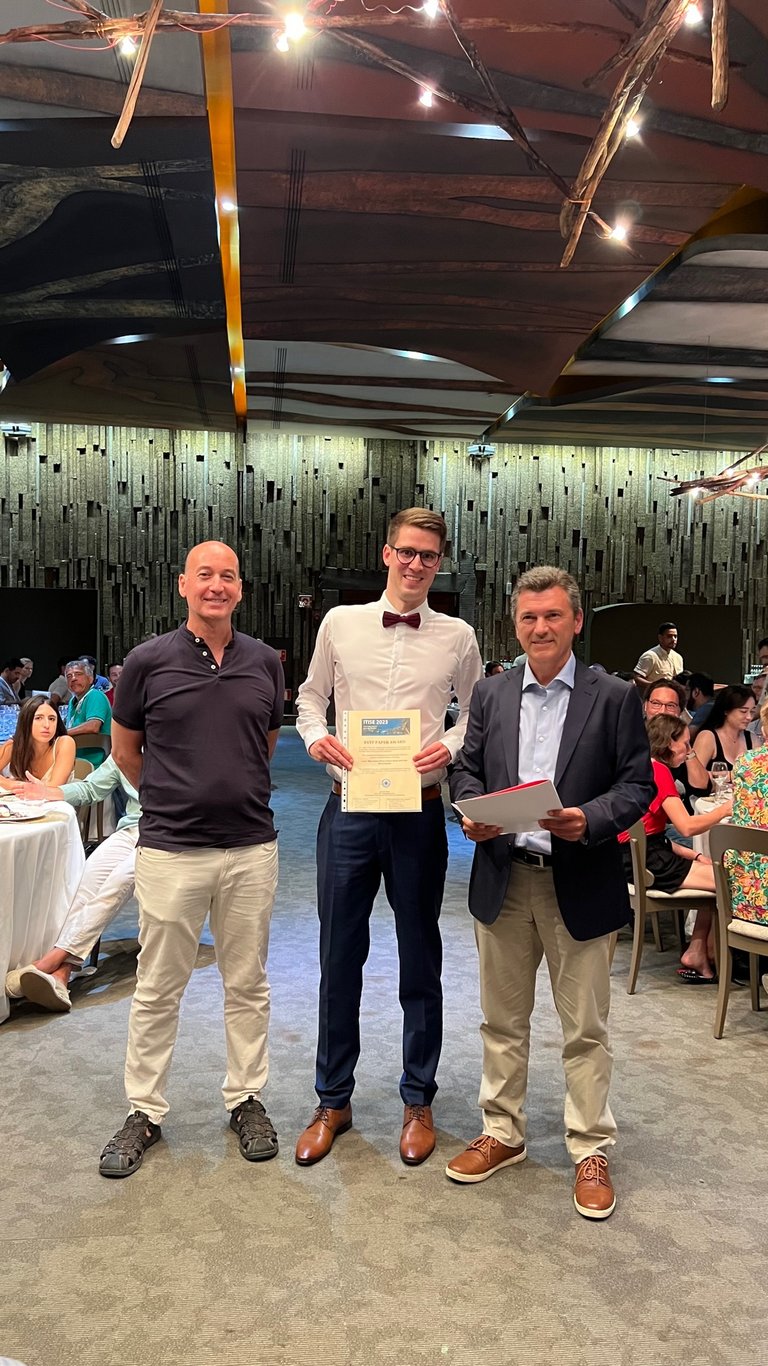
Data-driven modeling of complex relationships using artificial neural networks has been used successfully for many years and has now also arrived in everyday life: Artificial intelligence (AI) detects faulty parts in production, delivers print-ready translations, and finds the right movie for each person on streaming services, but the machine generation of accurate, robust, and explainable models still poses a challenge. While AI methods develop the basic strategies of machine learning, i.e., how input data is related to output variables, they do not explicitly specify the many parameters of a model. Instead, the algorithm independently "learns" the parameters of the model from the data provided, thereby continuously improving the estimates of the target variables.
View into the Black Box
This approach leads to increasingly precise estimates, more appropriate recommendations or more accurate translations. However, due to the black-box nature of the models, the decisions that these methods make independently are difficult to understand even for experts. This is not critical for many applications such as recommendation systems on online platforms or translation programs. Especially for safety-critical applications, such as in medicine, autonomous driving or energy technology, non-transparent, erroneous AI-based decisions can have serious consequences.
This is precisely where data analysis methods come in. In their work, the Ilmenau scientists used examples from energy technology to investigate various data analysis methods in order to optimize the performance and plausibility of computational models. Specifically, they focused on calculating the conductor rope temperature, determining network losses, and analyzing the vertical network load in the power transmission network, i.e., the electrical power delivered from the transmission network to the networks at lower levels and to the end consumers.
Simpler, better and more comprehensible learning models
By comparing different filtering methods as part of the feature selection data analysis, the researchers were able to identify the significant input variables for computing the target variables. This approach not only reduced the complexity of the machine learning models, but also significantly improved their performance. At the same time, it led to increased plausibility of the machine learning methods. "This not only highlights the strengths and effectiveness of data analysis as a pre-processing step in machine learning, but also contributes to the development of trustworthy and reliable models," emphasizes co-author and department head Prof. Peter Bretschneider:
Such models are highly relevant especially in safety-critical areas such as the energy sector as well as for numerous other application areas.
The successful completion of this work was made possible by the cooperation between the Department of Energy Utilization Optimization and 50Hertz Transmission GmbH. The latter provided the necessary data for the investigations.
ITISE is a three-day conference hosted by the Universidad de Granada and was held for the ninth time in 2023. It serves as a forum for discussion of the latest ideas and insights in time series analysis and forecasting - topics that are extremely relevant to many disciplines. Although these disciplines vary widely in their applications, they exhibit remarkably similar methodological approaches.
About the Energy Usage Optimization Group
The Energy Usage Optimization Group researches how electricity, gas and heating/cooling can be provided and used in an ecologically and economically optimal way, taking into account the fluctuating availability of wind and solar energy and energetic flexibility and storage potentials.
To this end, it is investigating and developing new AI-based methods and procedures to predict energy requirements and fluctuating feed-ins, to optimize power plant deployment and storage operations, and to optimally adapt energy procurement to the changing framework conditions.
Contact
Prof. Peter Bretschneider
Energy Usage Optimization Group